The Trust Equation: Generative AI’s dilema
Trust in computers is now on the other side of its origins
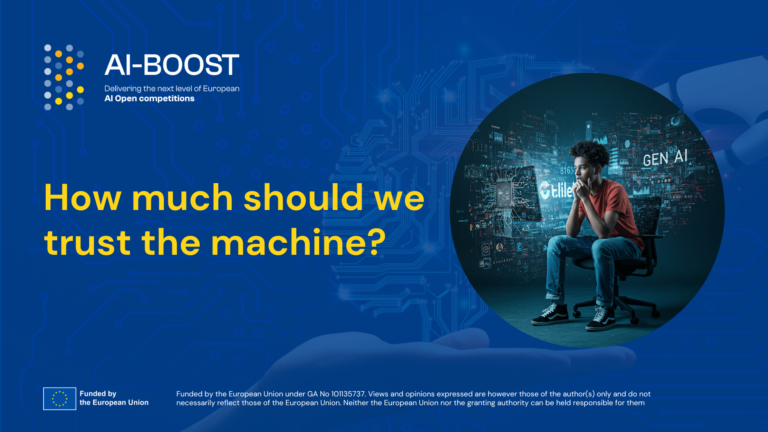
Computer inventors and manufacturers struggled to find a market and build customer trust. The UNIVAC was the first computer specifically designed for business applications, and the Remington Rand Company partnered with CBS (the TV chain) to explore ways to promote it. One of these was the live estimation of the presidential election results on 4 November 1952. The computer correctly estimated the surprising landslide that took Eisenhower to the presidency. However, the result was so disparate from expectations that the presenter hesitated to announce it. Nevertheless, once it was confirmed that computers were remarkably accurate, the perception of the capacity for precision and correctness shifted to what it is now: computers are infallible.
Many studies have demonstrated that humans today trust a computer’s response almost blindly. This potentially unjustified trust receives several names, such as “automation bias” and “machine heuristic”. Some of these beliefs extend to consider machines objective and impartial.
Until recently, machine learning was mainly concerned with learning deterministic models from data. Mathematically speaking, the goal was to obtain the best function from data for predictions on unseen cases or the future. That is, there is only one output for a given input. That is what is a machine. If you press some button and the machine does not do what it should do for that input, it is malfunctioning. In a sense, machine learning aimed to produce programs for computers that would make them behave like machines.
But now we have entered the world of generative AI, which could be considered the world of producing output indistinguishable form what could be in the dataset. Now, to the same input or prompt, we obtain different answers. Some of them would be entirely fabricated, incorrect, and misguided. They could be far-fetched from the facts in the data set. This could be fantastic for the creative industries, where the audience knows the story is fictional but has raised the colossal issue of fake-news, disinformation and its massive impacts in democratic societies. Citizens are acting on poor information that they now whole heartedly trust, as many effects combine to generate such misplaced trust.
It has been demonstrated that eliminating hallucinations of Large Language Models that include transformers is mathematically unsolvable. Using AI itself to exclude hallucinations has also been shown to be infeasible. Adversarial networks are precisely the technique by which the output of one is indistinguishable as fabricated or real by the other.
It is of concern that sources of news that want to maintain their prestige as trustworthy news, such as “El Pais” and “Le Monde” have partnered with Open AI to apparently eliminate misinformation. First, the size of the archives of these newspapers may be insufficient to create quality generative output, suggesting that pre-trained models with other data may already pollute the data set. Second, any data sets will produce biased models by deciding what documents were included in the data set or not. Not all the archives of “El Pais” and “Le Monde” are facts, there are also editorials with judgements and opinions. And finally, the nature of probabilistic construction from generative AI allows the possibility of fabrication. Generative AI is not a document or information retrieval tool.
The challenges for trustworthy AI start from reversing back our now huge faith on the output of computers.
* This reflective article was written by Vladimir Estivill-Castro, PhD, a project partner from Universitat Pompeu Fabra.
Follow AI-BOOST today to take part in the shaping of the next level of European AI open competitions.
Join AI-BOOST’s community on LinkedIn (@aiboost-project) & on Youtube (@aiboost-project)